Day 2 Summary of the 12 Days of OpenAI Live - Reinforcement Fine-Tuning
- Name
- Tison Brokenshire
Updated on
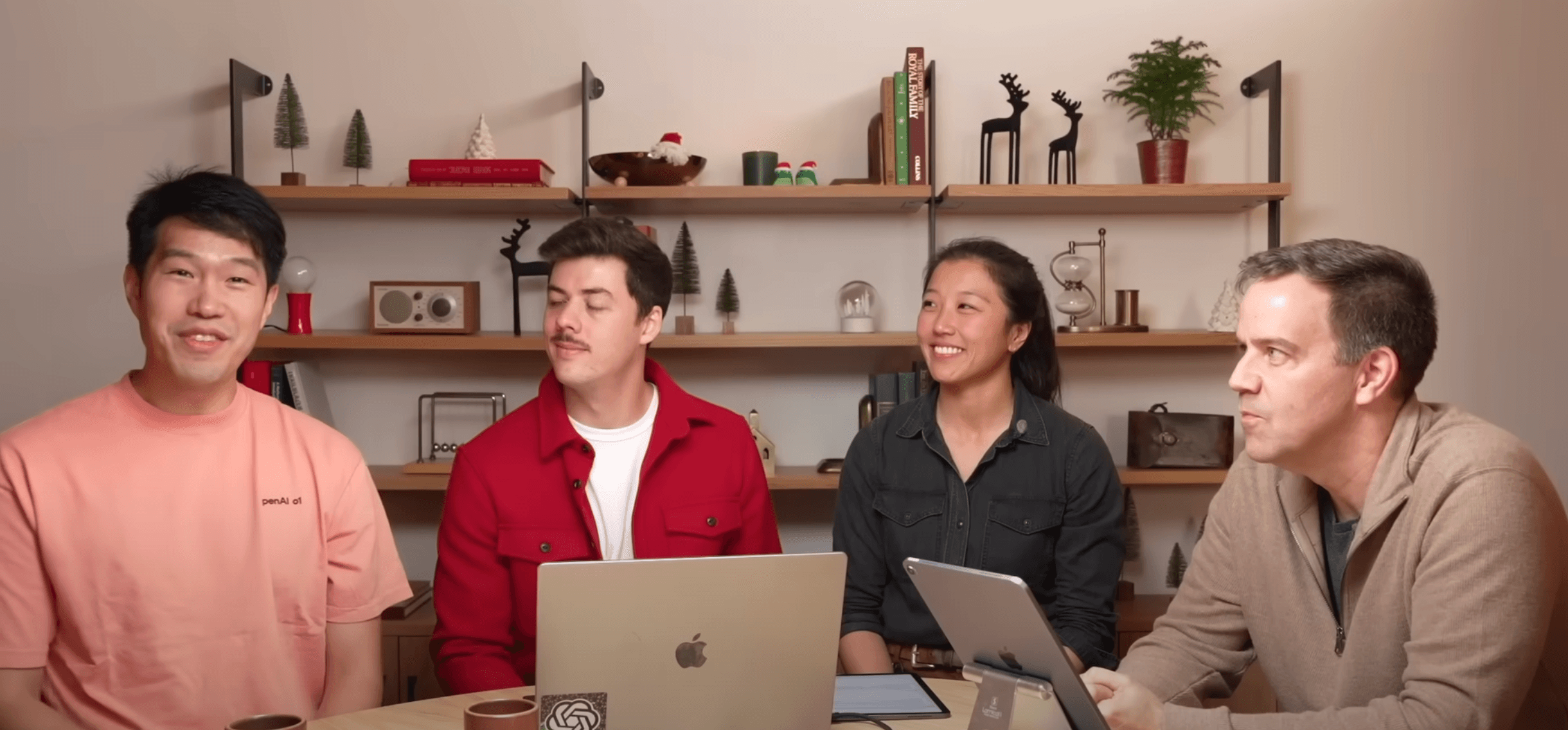
Following yesterday’s announcement of the O1 model’s general availability in ChatGPT—and its forthcoming introduction in the API—OpenAI has unveiled yet another breakthrough: reinforcement fine-tuning (RFT) for O1. On the second day of the “12 Days of OpenAI” live series, the research and engineering team showcased how reinforcement learning can now be applied directly by customers to customize O1 models for their highly specialized tasks. This marks a significant evolution in model customization capabilities, moving beyond conventional supervised fine-tuning toward a more dynamic, expert-level adaptation of large language models.
From Supervised Fine-Tuning to Reinforcement Fine-Tuning
Traditional supervised fine-tuning allows users to refine a model’s outputs by providing examples of desired responses and formats. This is perfect if you want to adjust a model’s style, tone, or surface-level behaviors. But until now, truly teaching a model to reason in new ways—especially within niche, complex domains—has remained challenging.
Reinforcement fine-tuning changes the game entirely. Rather than simply mimicking inputs, a reinforcement-fine-tuned model iteratively learns from feedback on its reasoning steps and outcomes. During the training process, the model:
- Takes time to reason through a problem.
- Produces a final answer.
- Is then “graded” by a custom evaluator (a “grader”) to determine how correct or useful that answer was.
Armed with this feedback, the model reinforces the reasoning pathways that lead to correct outcomes and suppresses those that lead to errors. The result is a model that can develop genuinely new reasoning strategies with as few as a few dozen examples—something not possible with standard supervised fine-tuning.
Bridging General Intelligence and Deep Expertise
The O1 series of models was introduced with a new capability: internal reasoning chains. O1’s improved architecture lets it “think” more deeply before arriving at a final answer. Now, with reinforcement fine-tuning, organizations can amplify this internal reasoning to solve their domain-specific problems more effectively.
Whether you’re in law, finance, insurance, engineering, or scientific research, reinforcement fine-tuning can help transform O1 into an expert assistant that excels in your particular field. As was highlighted, these techniques have already been put into practice:
-
Legal Analytics: OpenAI partnered with Thomson Reuters to customize O1 Mini (a smaller variant of O1) for legal analyses within their CoCounsel AI platform. Reinforcement fine-tuning allowed the model to navigate highly specialized legal reasoning tasks more confidently and accurately than ever before.
-
Biomedical Research: Justin Reighard, a computational biologist at Berkeley Lab, joined the live demo to illustrate how reinforcement fine-tuning can advance research into rare genetic diseases. The data used in this project originated from curated medical case reports. By scoring the model’s ability to identify disease-causing genes from patient symptoms, the research team guided the O1 Mini model’s reasoning toward better diagnoses—even outperforming the larger and more powerful O1 model.
A Closer Look at the Process
OpenAI demonstrated the end-to-end pipeline live:
-
Preparation of Training and Validation Data:
The team uploaded a curated dataset of about 1,100 medical case reports describing patient symptoms and known genetic causes. Crucially, the validation data contained diseases and genes not present in the training set, ensuring the model needed true generalization—not just memorization. -
Defining a Custom Grader:
A grader is a small piece of logic that scores the model’s outputs. For the biomedical use case, the grader compared the model’s proposed genes against the known causative gene. Answers closer to the correct gene earned higher scores. This tight feedback loop allowed reinforcement algorithms to shape the model’s reasoning pathways effectively. -
Kicking Off a Reinforcement Fine-Tuning Job:
After setting up the dataset and graders, the team initiated a training run on OpenAI’s infrastructure. Reinforcement fine-tuning leverages the same underlying techniques used internally at OpenAI to produce top-tier models like GPT-4 and the O1 series. Within hours to days, the process yields a specialized model finely tuned for the given task. -
Measuring Performance Gains:
The results were striking. Before reinforcement fine-tuning, O1 Mini could identify the correct causative gene as the top candidate about 17% of the time. The best O1 base model improved that to 25%. After reinforcement fine-tuning, O1 Mini leapt to 31%, surpassing the previously stronger O1 baseline. This improvement is not just a numeric bump—it represents the model genuinely learning new reasoning strategies and applying them to previously unseen cases.
Implications and Future Directions
Reinforcement fine-tuning heralds a new era of building domain-expert AI. By steering models with a combination of carefully chosen training data and a specialized grading system, organizations can create AI tools that handle complexity, uncertainty, and expertise-driven reasoning with remarkable proficiency.
-
Healthcare and Research: As demonstrated, researchers can push beyond surface-level analysis and develop AI assistants capable of nuanced diagnostic reasoning. With just a handful of examples, these models can start to emulate expert-level problem-solving, propelling scientific and medical research forward.
-
Legal, Financial, and Industrial Applications: Any domain where complex, expert-level reasoning is required—analyzing contracts, understanding intricate regulations, or performing high-stakes engineering calculations—stands to benefit from reinforcement fine-tuning. Models can become more accurate advisors, reducing time, cost, and error rates in highly specialized workflows.
Alpha Program and Public Launch
While reinforcement fine-tuning is not yet publicly available, OpenAI is opening up its alpha program to select universities, research institutions, and enterprises. The goal is to gather feedback, refine the product, and ultimately make reinforcement fine-tuning broadly accessible early next year.
For experts working on intricate tasks and eager to test this new customization approach, the alpha program offers a head start. If you meet the criteria—complex tasks, expert teams, and a desire to bring AI-driven reasoning into your domain—OpenAI encourages you to apply.
Looking Ahead
Day 2 of the “12 Days of OpenAI” ended on a high note, leaving us with a tantalizing glimpse of what’s next. Reinforcement fine-tuning transforms how we view model customization: from refining surface-level output to re-architecting the reasoning pipelines within a model’s mind.
As the countdown to the public release of reinforcement fine-tuning begins, the real question is not just what O1 can do, but what you can do with O1. From healthcare diagnostics to legal reasoning and well beyond, the applications are limited only by the imagination—and the expertise—of the community shaping them. Stay tuned as OpenAI continues to roll out more capabilities, demos, and insights over the coming days.